While machines are excellent at operating with and understanding structured data (such as spreadsheets and database tables), they’re not so great at deciphering unstructured data, for instance, raw text in English, Polish, Chinese, or any other human language.
To bridge this gap, Natural Language Processing is coming up with solutions. It allows the computers to know the meaning of specific concepts and how human beings use language when we talk or type – uncertainties, languages, grammar mistakes, shortcuts, accents, personal characteristics, and more. When we train machines to understand unstructured human language text, they can read what users want when they transcribe or deliver a phrase and obtain important and relevant data.
NLP is the key reason behind several applications we use in our routine life. Personal assistants, spell checkers, translation applications, and search engines- most of the app that has human language uses NLP algorithms so that machines can use and understand the words of people.
The evolution of natural language processing
In the 1940s, NLP appeared as machine translation (MT). That technology intended to solve Russian code in World War II, but initial efforts were defeated.
NLP took a step forward by launching SHRDLU, a language platform, and ELIZA in the 1960s. It is an advanced chatbot that could reproduce limited conversation based on a substitution methodology and pattern matching.
Till the 1980s, several systems were based on complicated hand-written rules, but NLP was transformed by adding machine learning algorithms for language processing. These advances made the source of what NLP is now.
By the end of the 2010s, deep learning came into the picture. It was Launched in the area of artificial intelligence once more and provided NLP to deliver radically enhanced results.
What are the benefits of NLP?
The large amounts of data businesses have at their disposal in the digital era is a source of the treasure of priceless business insights. Now companies can get maximum value for their data through intelligent NLP methods.
Examine text data in productive new methods
NLP allows businesses to convert textual data spread over the web into actionable business insights and advance the competition from tracking negative reviews to keeping up to date with the latest social media trends.
Tap hidden patterns
Practice topic modeling techniques to identify customer trends or hidden patterns in big amounts of unstructured text, including job applications, customer reviews, social media profiles, or emails.
Automate conclusions based on text input
Simplify your processes by including techniques like text classification to automatically analyze sentences, phrases, or entire documents into predefined collections.
Visualize text data
Convert thick walls of text data into powerful and eye-catching visuals and never crave the next big trend running your industry or company.
How does NLP work?
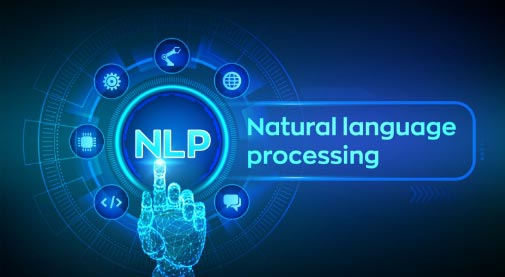
Machine learning is a field that deals with natural language processing. Like any other sort of data, complex systems store and analyze words and how they come together. Machine learning engines are given phrases, sentences, and even entire books. These messages are then analyzed using grammatical, syntactic, and other language norms and people’s natural speaking patterns. Then the system applies this data to detect patterns.
Syntactic analysis and semantic analysis are the main techniques used in Natural Language Processing. The arranging of words and phrases to construct well-formed sentences that make grammatical sense is syntax. Syntactic analysis is a type of NLP that evaluates how natural language conforms to a language’s grammatical (and other) norms. Semantics, on the other hand, is the study of the meaning transmitted in a text. The semantic analysis entails using computer algorithms to comprehend the data.
Syntactic and semantic analysis approaches are used together to allow computers to read text or listen to the voice, parse words, measure sentiment, and determine which sections are significant. Today, NLP software can analyze more language-based data in a shorter amount of time than humans can, and it can do it consistently and fairly without tiring. And this has far-reaching consequences for corporations.
What is NLP used for?
Chatbots
With natural language processing, your chatbots can be performed to seem more social and improve your customers get the knowledge they need immediately, improving customer experience.
Voice systems
Fuse NLP systems to understand voice commands and elevate customer interactions with your service and brand to a higher level. Although NLP is based on text, a ‘voice system’ practices speech recognition to use recognized speech into text and then explains this text based on NLP. Speech recognition and NLP are usually used concurrently, for instance, in Siri and Alexa.
Sentiment analysis
Need to understand whether tweets about your business are great or bad so that you can ask your customers’ interests? Sentiment analysis utilizes NLP to assist businesses in understanding what’s being told regarding them on the web and social media channels.
Battling spam and managing inboxes
Spam detection practices Natural Language Processing to prevent undesired emails and other information out of your inbox. NLP can also be utilized to sort messages from some contacts into separate portfolios.
Machine translation
Natural Language Processing is required if you wish to include a translation feature in your app. The difficult element of computer translation is preserving meaning rather than translating individual words. This is a difficult technical problem at the heart of NLP.
Advanced “conversational” search
When conducting searches, website users are human, and like all people, they occasionally forget important details, make spelling errors, mix up brands, or use slang or “conversational” terminology. All of these factors are taken into consideration by NLP, which connects the dots and produces accurate results that are both relevant and beneficial to the consumer.
Information extraction
Natural Language Processing can be used to automatically summaries extensive papers or extract relevant keywords for search purposes. These types of NLP applications are used in the legal business to help lawyers search through hundreds of pages of papers in legal disputes to identify essential information.
Which Companies uses NLP?
One of the most well-known examples of companies adopting NLP is Google Translate. Every day, half a billion people utilize the system to convert over 100 different languages. NLP is also used in the company’s voice search.
Amazon
NLP is used to power Alexa, Amazon’s smart speech assistant. The device can understand and respond to questions like “what’s on my schedule,” as well as carry out commands like “lower the lights.”
Coca-Cola
Ask Coca-Cola virtual assistant is introduced by one of the soft drink giants to help its customer service department and improve customer experience. The service now manages 30,000 consultations a month, hugely decreasing the number of inbound calls and building efficiency in customer service.
Wrapping Up
Automation can assist in quickly grow your business. When you develop a site’s navigation, make products easy to practice with support from chatbots, or develop services by examining analysis, your business stands to gain.
NLP makes it feasible to perform all those tasks and then some. The right software can assist you in taking benefit of this interesting and evolving technology. For a complete solution, check out how our AI solution supports many businesses to become more customer-centric.