Synopsis of Edge Computing
- The phrase “Edge computing” refers to the processing as an empowered worldview. It gives information about data nearer to the device or data reference.
- Edge Computing provides data created by IoT to be prepared near its source rather than transferring it to a great way to data centers or clouds. It’s connected to handling determined data near the data reference, which is deemed the ‘edge’ of the community.
- It’s connected to working applications as close as feasible to the site where the data is being made, preferably inducing together cloud or data gathering position.
- Edge computing was created due to the IoT solution’s significant improvement, which partners with the web to allow information from the cloud or transfer data following to the cloud.
Synopsis of Edge AI
- Edge AI is the system that uses AI/ML algorithms to prepare the data which is created by the hardware devices at the regional level.
- Edge AI means that the AI algorithm is introduced nearby on hardware devices and algorithms utilizing the machines’ data. They save the results nearby on the devices, and following that, the devices used for things are connected to the internet and transfer the knowledge to the cloud for processing and storage.
- Edge computing consists of limited computing devices optimized for Artificial Intelligence and quickly blended into the store. Edge computing is perfect for retail stores to prepare and examine the endless amount of video data and IoT sensors captured on-site in real-time.
Advantages of Edge AI
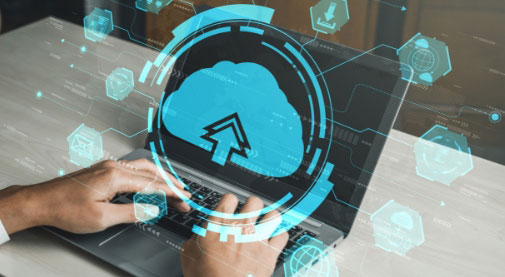
- Edge AI’s advantages are pace and can recognize the results by combining Smart IoT solutions and devices and functionality to use AI at the end for penetrations.
- Its versatility allows intelligent devices to help many businesses.
- Edge AI also gives high safety and assurance levels with improved protection features, and edge AI-powered devices help reduce this uncertainty.
- No different knowledge is necessary to complete the Edge AI-empowered devices. The device automatically gives vital insights on the spot within innovative graphical interfaces or consoles.
- It decreases expense and latency periods for an enhanced user experience. This helps integrate technologies centered on the user’s knowledge to communicate in real-time to make payments.
Drivers of Edge AI and Edge Computing
Edge computing Solutions is a shared computing paradigm that does required calculations and stores data closer to the device’s position. There is a difference that edge computing will substitute Cloud computing. On the opposite, it works in cooperation with the Cloud. Big data will ever be prepared on the Cloud. However, instant data produced by the users and partners only with the users can be measured and prepared on edge. There are various drivers of Edge Computing and Edge AI.
-
Latency
The obvious purpose for jobs to be performed on edge is latency. The suspension while transferring data to the Cloud for processing and then decisions are sent back over the network to a regional device. In some states, AI models must be prepared at the edge or at the machine to make decisions quickly without relying on network connectivity and driving massive data back and ahead.
-
Privacy
In unusual situations, distributing personal and sensitive data across borders has increased data privacy concerns. Here, AI on edge assists by only distributing the data that needs more evaluation, which reduces the volume of data given and decreases the possibility of break-in privacy.
-
Execution
AI models can process the data much faster on the machine than on the Cloud because it does not require moving back and forth. But there are still cases where data processing in the Cloud is more reliable. When decisions need substantial computational strength and do not require to be performed in real-time, AI should stay in the Cloud. For instance, In healthcare, when AI is used to describe an ECG while in agriculture to examine crop quality, data gathered by vibration over a field where one can expect a few minutes or a few hours for the conclusion is satisfactory to do this processing in the Cloud. Know more about AI MODEL DEVELOPMENT & TAGGING
-
Bandwidth
To build insights from AI, data requires to migrate to the Cloud. As connection speed changes in many sectors of the world, it is sometimes simple to carry data from/to the server from distant locations. However, AI at the edge works the difficulty by sending only the needed data for further study.
Inducing AI in Edge Computing
As we recognize the likely trends of AI services towards a prospect powered by the smart cloud and edge. They measure a massive system that the public cloud can use and are powered by AI for each application model.
The Edge AI is a constantly growing collection of connected systems and many devices that collect and examine data as per end-user.
Developing the volumes of the data across all the connections. The customer has been receiving some information about the versatility of sending AI capabilities in several conditions.
The customer can immediately review the data where the information includes communicating continuous bits of information that are very sensitive and relevantly careful of the administrations. That customer sends on their bases and doesn’t transfer the customer data model. The image or text that examines while delivering the AI on the cloud.
Summing-up
Edge Computing Solution is the fortune teller of Cloud Computing. This does not imply that one technology will substitute the other. Preferably, they will be matched so that the services’ public advantages produce without placing extreme loads on the network. Performing Edge AI in Edge Computing is versatile and empowers intelligent devices to help different industries.
Talk to our Artificial Intelligence Experts for developing cost-effective AI-Driven Solutions.