Humans will never be able to interpret the vast amounts of data collected by each production line. As a result, artificial intelligence will be necessary to make sense of all of this information. ML algorithms can uncover data patterns in structured and unstructured data sets that would be impossible for humans to perceive.
According to a McKinsey Global Report, “smart factories have the potential to produce as much as $3.7 trillion in value per year by 2025.”
With an AI-enabled smart factory, manufacturing can run at breakneck speed while lowering costs and improving customer satisfaction. Enterprises can prevent machine downtime by predicting failures, controlling inventories by keeping track of stocks, estimating projected delivery time, and providing the finest quality goods using Artificial intelligence solutions.
Let’s take a closer look at how Artificial intelligence solutions might assist manufacturers in achieving their smart factory goals:
Computer vision for quality control
Computer vision can monitor the manufacturing process and detect flaws such as minuscule fractures in machinery, unpredictable machine movement, and other minor imperfections that arise during production.
This is especially useful in additive manufacturing, where 3D printers may utilize high-resolution cameras to monitor pits, streaks, divots, and other patterns that aren’t apparent to the human eye. It may also be programmed with alignment, dimension, and measurement information to guarantee that the product is of standard sizes. Artificial intelligence can learn from video footage of the printing process and eventually identify product or process faults.
Generative design
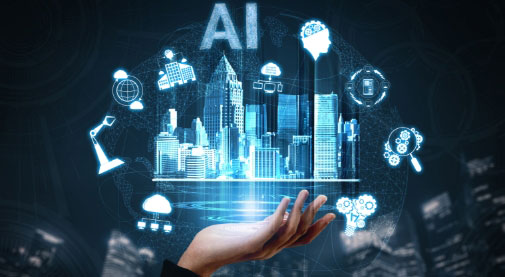
Artificial intelligence is transforming the way manufacturing businesses use generative design to create goods. It is an iterative design process in which comprehensive design information is fed into AI algorithms as input. Several design elements, such as manufacturing techniques, product material type, time restrictions, and budget limits, may be covered in this data. Considering all of these variables, the algorithm will examine every potential variation of a solution and return a list of the best options as an output.
The product designer can also establish a minimum and maximum limit to guarantee that the algorithm generates numbers within the given range. Proposed solutions are presented as outputs, which may be further examined using machine learning to determine whether the design fits the requirements. This procedure can be repeated until the best design option is discovered.
Assembly Line Integration & Optimization
Manufacturers utilize a variety of equipment, all of which send a variety of data to the cloud. However, all of these diverse forms of data do not operate together on the cloud, making it difficult to glean business insights. To gain a complete overview of the manufacturing activities, you could need a dozen dashboards and a team of subject matter experts.
You may ensure that you have a bird’s-eye view of all activities by developing an integrated application that can extract data from all the IoT-connected devices in your ecosystem.
Furthermore, on top of all the data insights, adding Artificial intelligence solutions to your IoT network may assist automate the assembly process. When one of the assembly line’s equipment fails, for example, the production supervisor is told. If equipment fails, the system creates a contingency plan and reorganizes activities automatically.
Predictive maintenance
Furthermore, on top of all the data insights, adding artificial intelligence to your IoT network may assist automate the assembly process. When one of the assembly line’s equipment fails, for example, the production supervisor is told. If equipment fails, the system creates a contingency plan and reorganizes activities automatically. Manufacturers may now use predictive maintenance to eliminate equipment breakdowns thanks to Artificial intelligence solutions.
Asset usage data may be put into machine learning models to anticipate future component breakdowns in predictive maintenance. This method will prevent the problem from occurring, resulting in a mostly unbroken production process. Because it focuses on optimizing asset life, predictive maintenance outperforms reactive and preventive maintenance and utilization. Furthermore, the equipment or components are only maintained when they are likely to break, ensuring that no money is wasted on perfectly functional parts.
Digital twins
Manufacturing organizations can utilize digital twin technology to produce a virtual image of their production facility, goods, or machine components that duplicate their physical properties. The digital twin can reflect real-time information about the actual environment by utilizing cameras, sensors, and other data collecting systems. To put it another way, a digital twin can generate a live model of the factory plant.
According to Gartner, “By 2021, 50% of big industrial enterprises will deploy digital twins, leading in a 10% boost in effectiveness for such organizations.”
Combining the digital and physical worlds allows for proactive issue management by monitoring production facilities and evaluating data. Manufacturers may use digital twins to consider scenarios that might enhance asset performance and forecast machine faults, which can aid decision-making. The digital twin can help firms better understand their goods by displaying their performance in a production setting and allowing workers to utilize it in real-time. This virtual-world information may then be used in the actual world to alter product design and build future goods.
Teksun has developed tailored solutions for manufacturers to automate their facilities and make their operations more effective while keeping costs down as part of our machine learning services. Get in contact with us if your company wants to progress toward smart manufacturing or Industry 4.0.